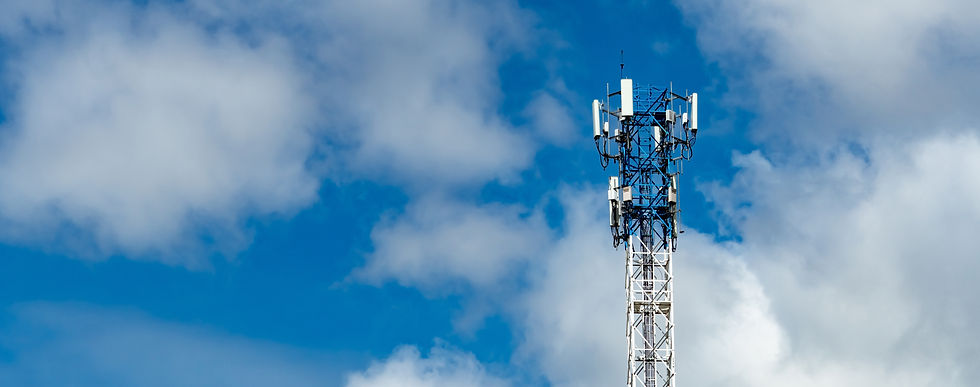
Letting Data Speak!
Case Study
Optimizing Alarm Resolution for Telecom Provider

About the Client
A leading telecom company providing a wide range of services including mobile and fixed-line telephony, broadband internet, operating an extensive network infrastructure supporting millions of customers across various regions.

Challenge
The client faced operational inefficiencies due to frequent alarms triggered by router downtimes, leading to delayed resolution times and potential impact on service delivery and customer satisfaction. They needed to investigate root causes, gain insights from alarm data, develop predictive capabilities, and implement real-time alarm correlation for efficient management and resolution.
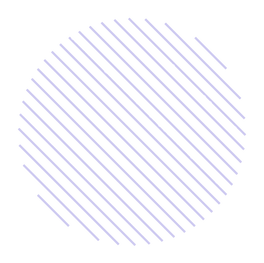
Key Results
Reduced the number of alarms to be reviewed for resolution by 80%
Enhanced prediction accuracy of alarm causes by 65%
Implemented real-time alarm correlation, reducing response times by the Network Operations Center (NOC) team by 60%
Solution
The project implemented a comprehensive approach to optimize alarm resolution:
Conducted thorough root cause analysis of historical alarm data related to router downtimes
Performed Exploratory Data Analysis (EDA) to identify critical time factors and patterns in alarm triggers
Developed a probabilistic model to predict causes of specific alarm types, enhancing prediction accuracy
Implemented an Airflow Directed Acyclic Graph (DAG) for continuous model learning and refreshing
Integrated processed data into a database for real-time access by the Network Operations Center (NOC) team via a GUI
Created a PySpark script for real-time alarm correlation, enabling swift identification and resolution of issues

Technologies Used
PySpark
Airflow
Statistical modeling techniques
Data visualization t
Other Case Study Items
AI Model for Retail Shelf Monitoring
JashDS revolutionized retail shelf management for a major grocery chain by developing an AI-powered real-time monitoring system. The solution utilized advanced computer vision techniques and deep learning models to detect out-of-stock and misplaced products, significantly improving inventory accuracy and enhancing the customer shopping experience while reducing manual labor costs.
AI-Driven Candidate Screening Revolution
JashDS revolutionized a company's hiring process by developing a GenAI-powered candidate screener that reduced time-to-hire by 50% and improved hiring outcomes. The solution leverages advanced language models to conduct dynamic, role-specific interviews, automatically generating and adapting questions based on job descriptions and candidate responses.
AI-Powered Resume-Job Matching Engine
JashDS developed an AI-powered resume-job matching engine for a leading Indian job portal, processing over 1M resumes and 50k job descriptions. The solution employed BERT embeddings and HNSW algorithms to create personalized job recommendations for candidates and streamline resume screening for recruiters, significantly enhancing the job search and recruitment processes.